ミュンヘン工科大学、Toyota Motor Europe(トヨタ モーター ヨーロッパ株式会社)、ウーブン・バイ・トヨタの研究者らは12月17日(火)、スマホなどの汎用デバイスで撮影した短い単眼映像から3D Gaussian splatsで頭部アバターを再構成し、新規ビューにアニメーションとフォトリアルなレンダリングを行う技術「GAF(Gaussian Avatar Reconstruction from Monocular Videos via Multi-view Diffusion)」を発表した。
We propose a novel approach for reconstructing animatable 3D Gaussian avatars from monocular videos captured by commodity devices like smartphones.
Photorealistic 3D head avatar reconstruction from such recordings is challenging due to limited observations, which leaves unobserved regions under-constrained and can lead to artifacts in novel views.
To address this problem, we introduce a multi-view head diffusion model, leveraging its priors to fill in missing regions and ensure view consistency in Gaussian splatting renderings.
To enable precise viewpoint control, we use normal maps rendered from FLAME-based head reconstruction, which provides pixel-aligned inductive biases.
We also condition the diffusion model on VAE features extracted from the input image to preserve details of facial identity and appearance.
For Gaussian avatar reconstruction, we distill multi-view diffusion priors by using iteratively denoised images as pseudo-ground truths, effectively mitigating over-saturation issues.
To further improve photorealism, we apply latent upsampling to refine the denoised latent before decoding it into an image.
We evaluate our method on the NeRSemble dataset, showing that GAF outperforms the previous state-of-the-art methods in novel view synthesis and novel expression animation.
Furthermore, we demonstrate higher-fidelity avatar reconstructions from monocular videos captured on commodity devices.
私たちは、スマートフォンのような汎用デバイスで撮影した単眼映像からアニメーション可能な3D Gaussianアバターを再構成するための新しいアプローチを提案します。
こうした、録画からフォトリアリスティックな3D頭部アバターを再構成することは、撮影された範囲が限定されていることから困難です。撮影されていない領域が不自然な状態のままとなり、新しいビューにおいてアーティファクト(データの誤り)を引き起こす可能性があります。
この問題に対処するため、私たちはマルチビュー頭部拡散モデルを導入し、その事前分布を活用して欠損領域を埋め、3D Gaussian splatsのレンダリングにおけるビューの一貫性を確保しました。
そして、正確な視点制御を可能にするために、FLAME(Learning a model of facial shape and expression from 4D scans)ベースの頭部の再構成データからレンダリングしたノーマル(法線)マップを使用し、整列したピクセルの帰納バイアス(※学習アルゴリズム)を提供します。
また、入力映像から抽出したVAE(Variational Auto-Encoder、変分オートエンコーダー)の特徴に拡散モデルを条件付けし、人物の顔の同一性と外観のディテールを保持します。
3D Gaussianアバターを再構成するために、反復的にノイズ除去された画像を擬似的なグランドトゥルース(機械学習モデルのトレーニングに用いる基準データ)として使用することで、マルチビュー頭部拡散モデルの事前評価を抽出し、過飽和の問題を効果的に緩和します。
そして、画像のデコード前にアップサンプリングを適用してノイズ除去を実施した情報を洗練することで、フォトリアリズムをさらに改善します。
本手法をNeRSembleデータセットで評価した結果、GAFは新規ビュー合成と新規表情アニメーションにおいて、従来の最先端手法を凌駕することが示されました。
さらに、汎用デバイスで撮影された単眼動画から、より忠実なアバター再構成を実証します。
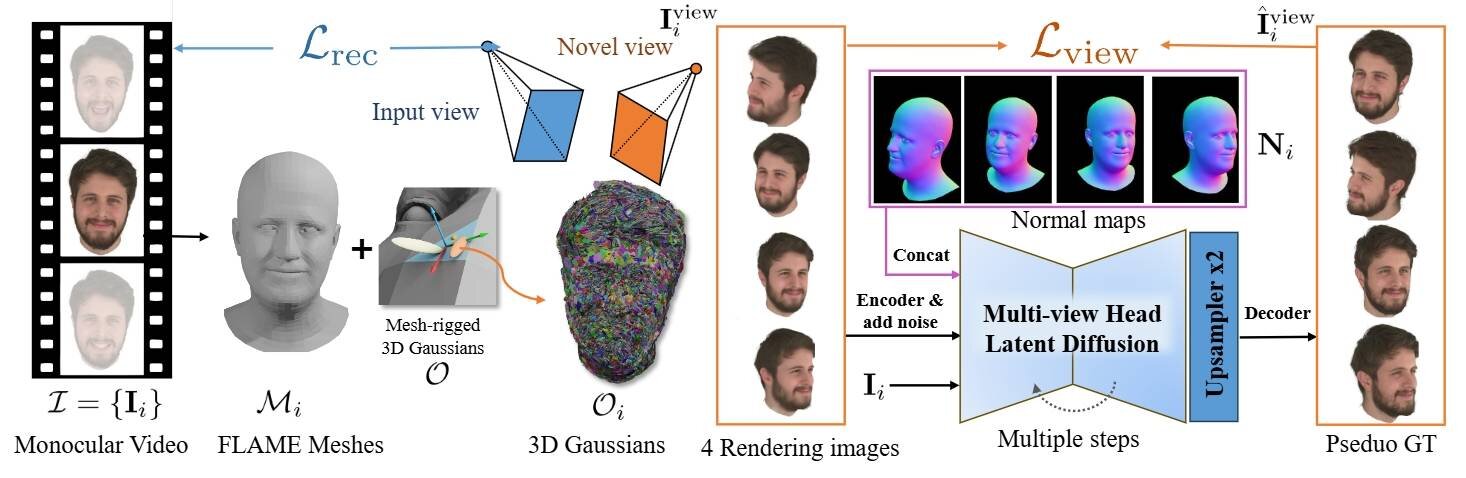
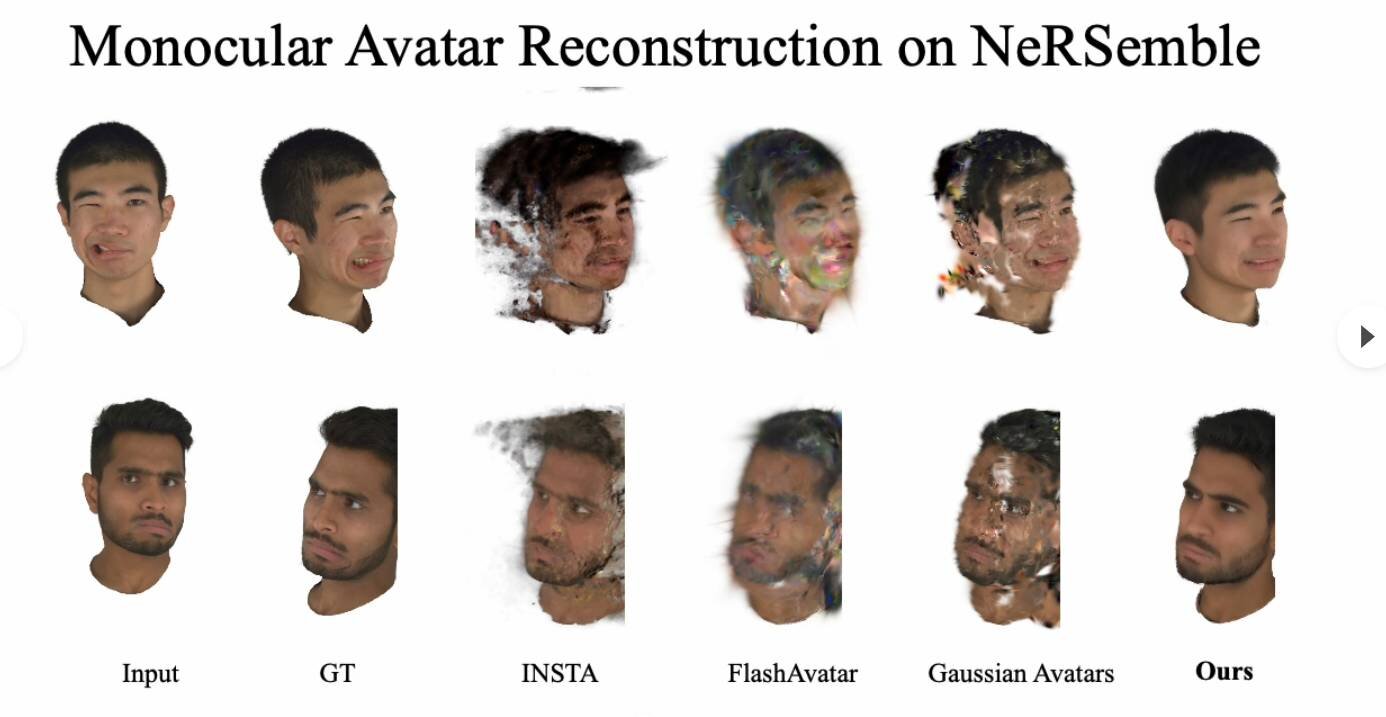
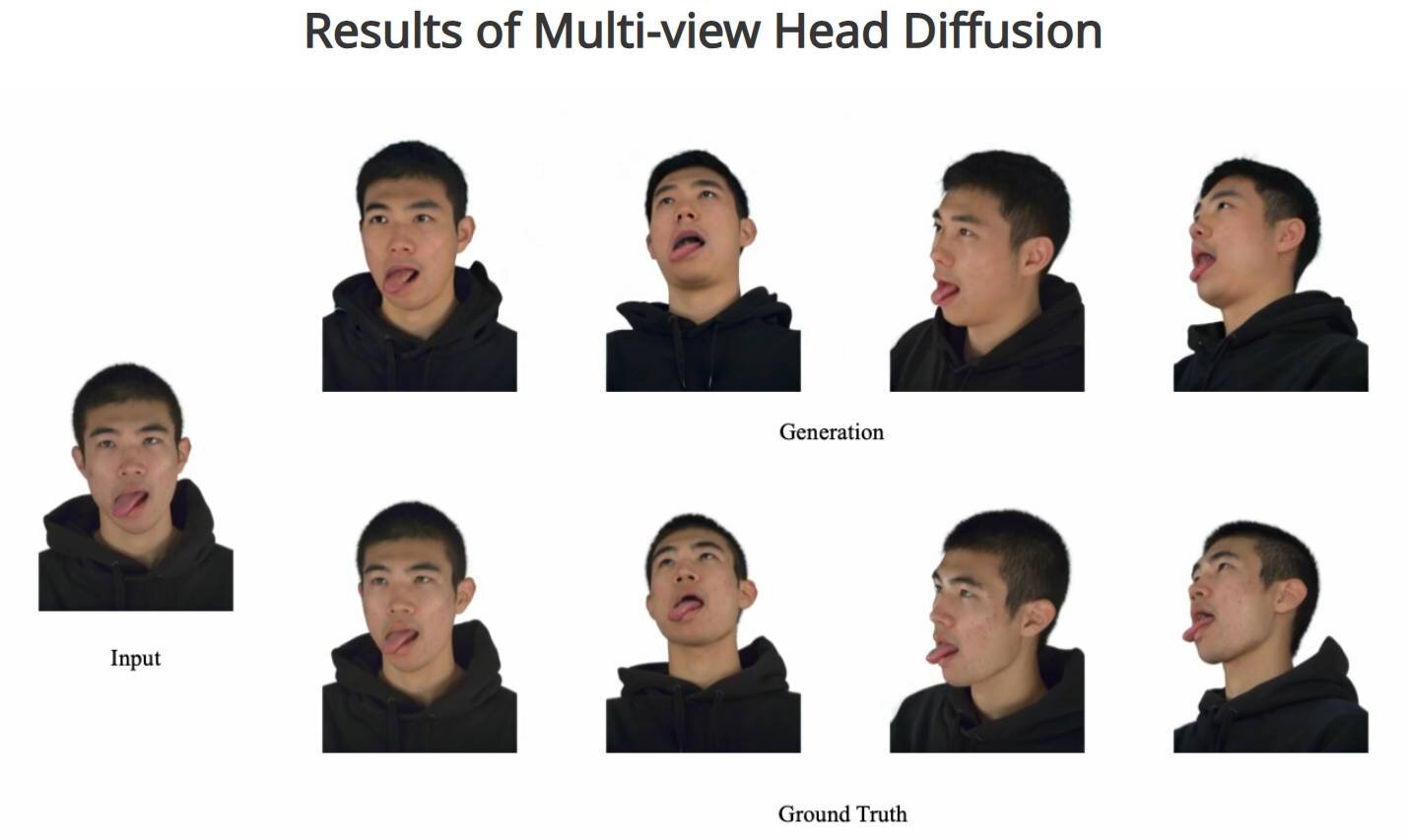
■GAF: Gaussian Avatar Reconstruction from Monocular Videos via Multi-view Diffusion(公式プロジェクトページ、英語)
https://tangjiapeng.github.io/projects/GAF/
■GAF(GitHub)
https://github.com/tangjiapeng/GAF
CGWORLD関連情報
●Niantic、3D Gaussian splats用の新ファイルフォーマット「.SPZ」発表! オープンソースかつコンパクトな仕様でユニバーサルフォーマットを目指す
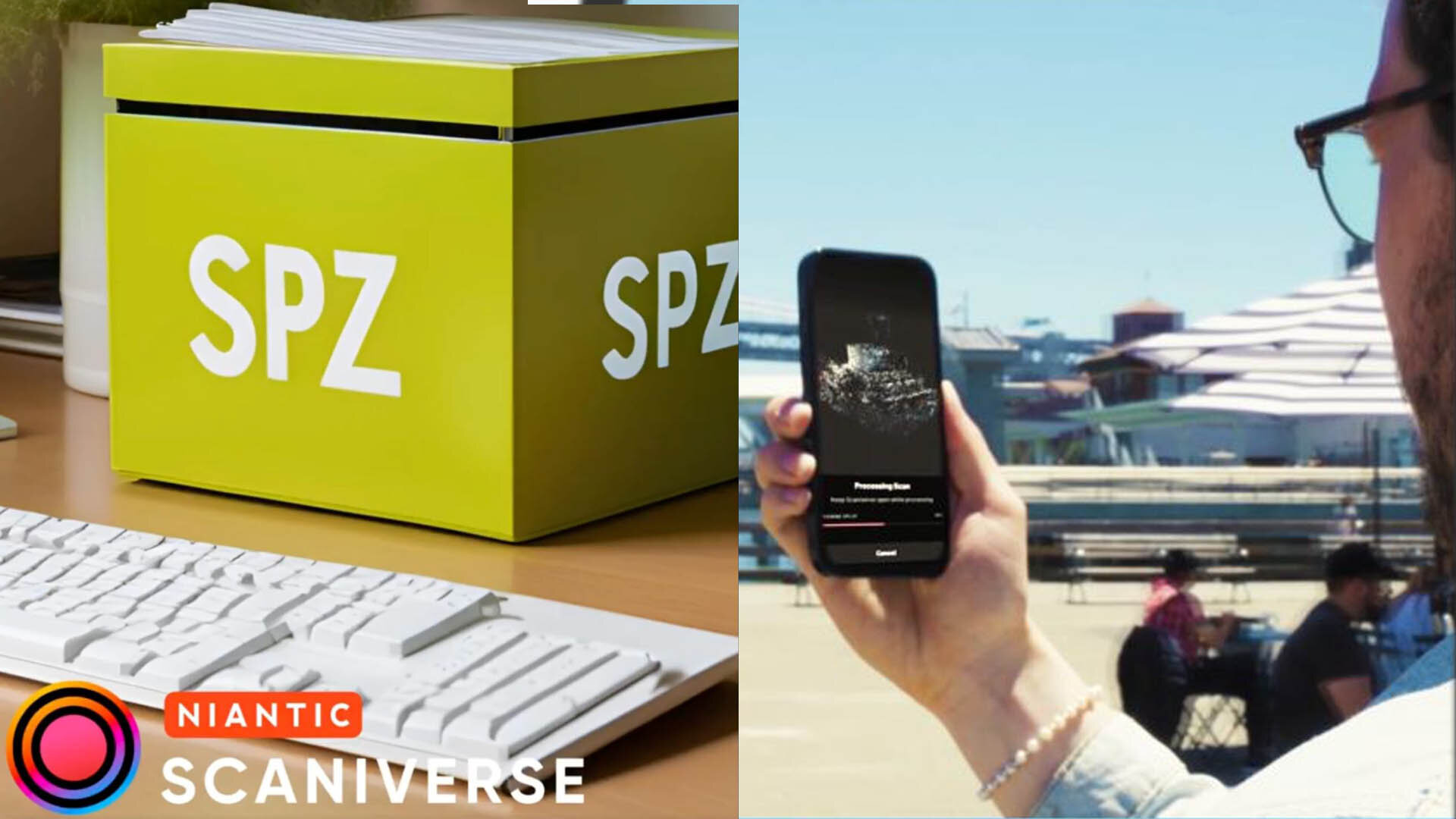
Niantic社は、ボリュームレンダリング手法の3D Gaussian splats用の新しいファイルフォーマット「.SPZ」を発表した。現在の主流である「.PLY」形式よりも低容量でメモリ消費も少ないコンパクトな仕様で、業界標準を目指すという。GitHub上でオープンソース(MITライセンス)として公開されている。
https://cgworld.jp/flashnews/202411-Niantic-spz.html
●NeRFとGaussian Splattingで画像/動画から3Dシーンを生成できる「Postshot v0.4」リリース! 品質向上、カメラターゲットのトラッキング、HDR映像のトレーニングなど
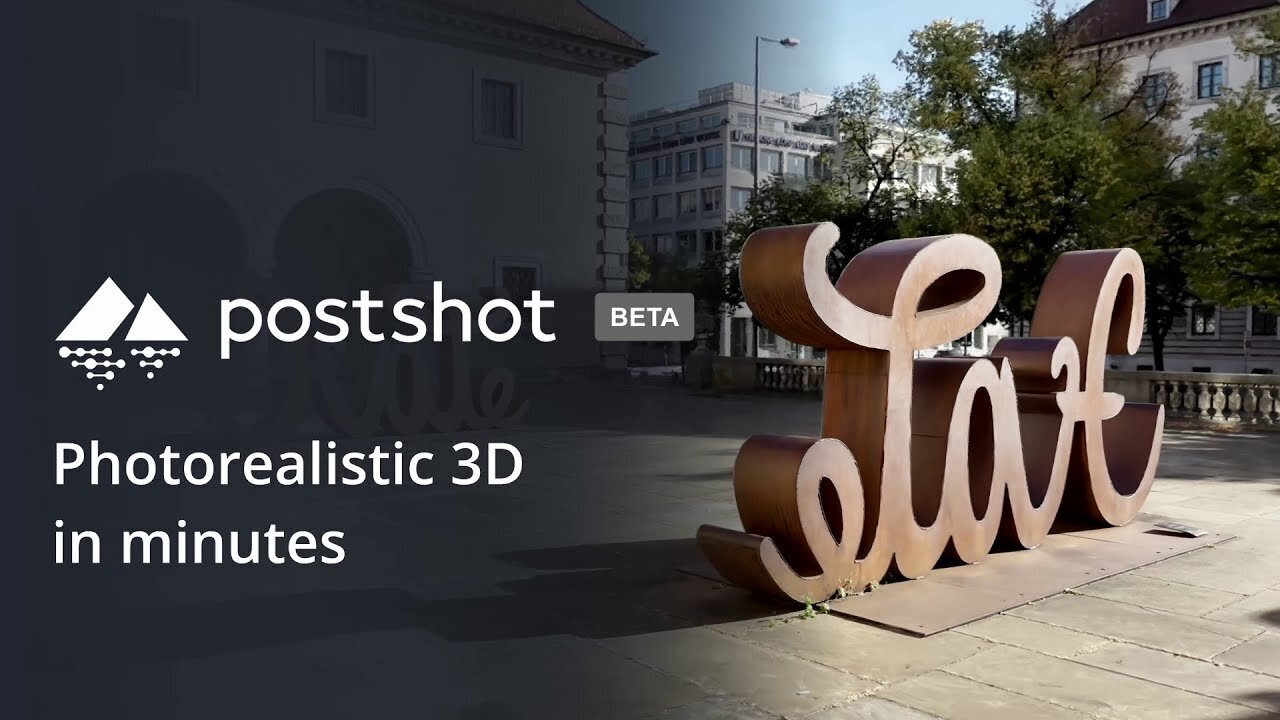
Jawset Visual Computing社は、3D Gaussian SplattingとNeRF(Neural Radiance Field)技術を使用して、画像または動画から3Dシーンを生成するツール「Postshot v0.4」をベータ版として公開。動作環境はWindows 10以降、GeForce RTX 2060以降またはQuadro T400/RTX 4000以降。現在は無料で利用できる。
https://cgworld.jp/flashnews/202408-Postshot.html
●機械学習を使った新しいレンダリング技術3D Gaussian Splattingを扱えるオープンソースのツール「SuperSplat 1.0」リリース! ブラウザから利用可能
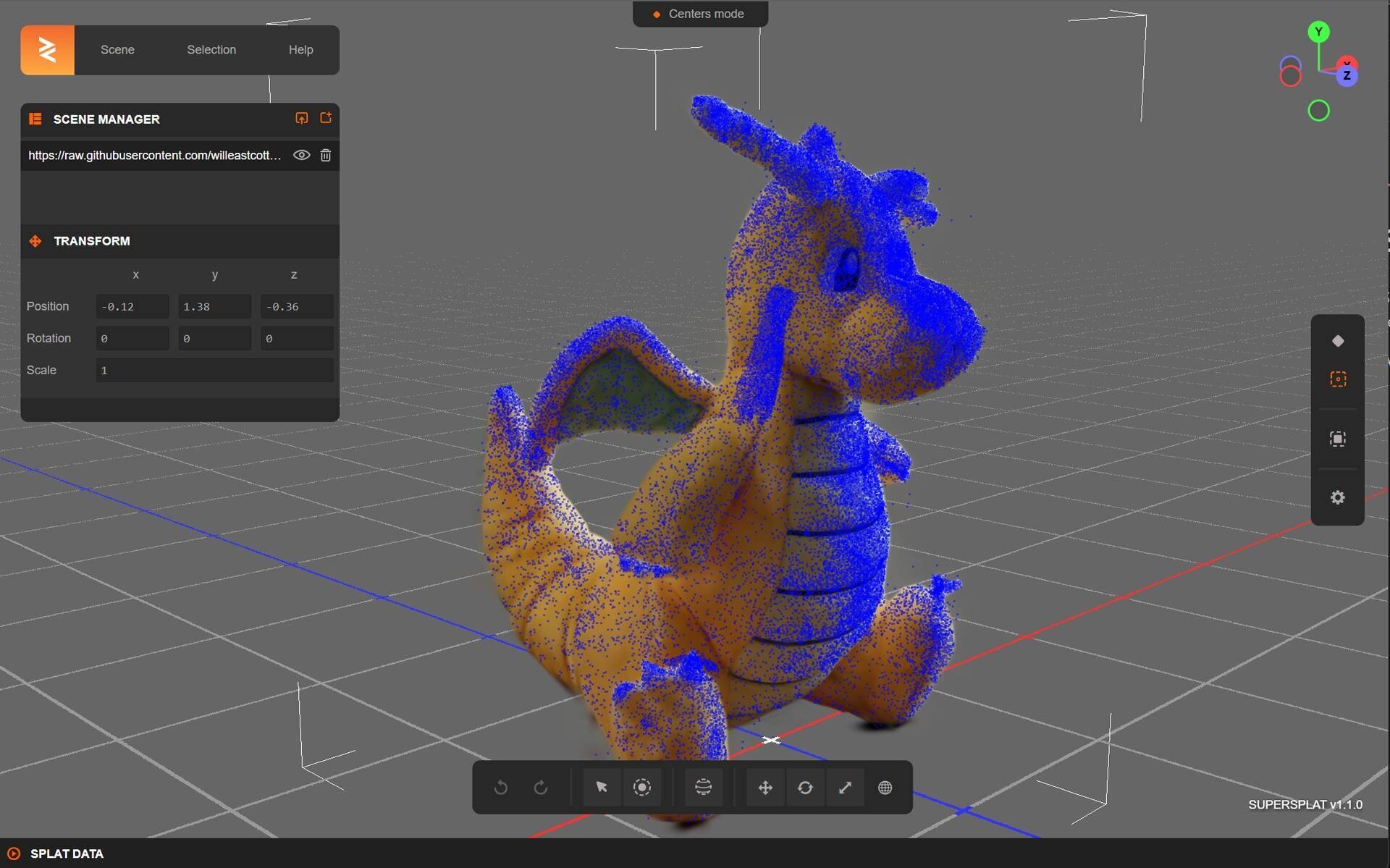
Playcanvas社は、3D Gaussian Splatsの編集&最適化ツール「SuperSplat 1.0」をリリース。Webブラウザベースのオープンソースツールで、キャプチャしたデータを手軽に最適化し、PLY形式などで保存できる。
https://cgworld.jp/flashnews/202408-SuperSplat.html